Ever tried to piece together a jigsaw puzzle with missing pieces? That's the frustration of sifting through messy data—where what is data cleansing becomes your lifeline. Imagine diving into an ocean of numbers and coming up for air clutching pearls of insight—that's the power of clean data.
We've all been there, right? Knee-deep in spreadsheets only to find that crucial figures are playing hide and seek. It's like planning a road trip but realizing your map is from 1995; you need current, accurate info to reach your destination smoothly.
You're about to embark on a journey through datasets scattered with duplicates, riddled with errors as common as potholes in springtime. But what if I told you that by the end we'll turn chaos into clarity?
The best part? You'll learn how pristine the process is and get to experience firsthand the benefits of a well-organized system. We're excited for you to start this journey with us and learning about what is data cleansing.
Data quality is a big concern for data migration projects. Cleaning data isn't exactly a glamorous job, but if you remove irrelevant data from your environment, and remove duplicate data, your data migration project might be more successful.
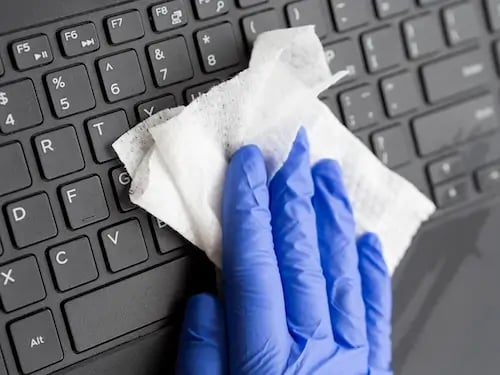
Table Of Contents:
What is Data Cleansing in Modern Business
Data cleansing isn't just a buzzword; it's the lifeblood of savvy decision-making. Think about it—dirty data is like having a GPS that leads you to all the wrong places, while clean data sets your business on the path to success. It's simple: if your dataset has more duplicates than an echo chamber or more structural errors than a house of cards, you're in for trouble.
Now let’s talk specifics. Tackling these gremlins head-on means less time chasing wild geese and more time hitting those sweet spots of opportunity. For instance, did you know that an effective data cleaning tool can sweep away unwanted outliers as easily as crumbs from a table? Or that with just some basic data entry discipline, we can prevent human error from throwing wrenches into our finely-tuned machines?
Many organizations have gathered data through data entry systems for years. Errors in data points, missing data, incomplete data and human error have all led to potential issues that affect data quality in your business.
Cleanliness might be next to godliness but when it comes to datasets—it's where profits hang out too. So roll up those sleeves and start scrubbing because every missing value filled and irrelevant observation axed sharpens your competitive edge.
Identifying and Rectifying Common Data Errors
The precise nature of data in every organization is different, but it's clear that data quality is essential in making good decisions. If your data quality is generally poor, and you're basing decisions on that, then it's obvious that the ultimately the decisions are also likely to be poor.
Let's look and some common data entry errors.
Tackling Structural Errors for Improved Data Integrity
Data integrity is the backbone of reliable analysis, yet structural errors often throw a wrench in the works. Think about it: if your data's structure is as unpredictable as weather forecasts, you're bound to run into trouble. Correcting incorrectly formatted data can feel like untangling headphones—frustrating but necessary. It starts with setting clear naming conventions and validating against them consistently.
Imagine handling outliers that don't fit any logical pattern; they stick out like sore thumbs. Robust data cleaning tools can help to spot these oddballs quickly because even one outlier can skew your entire analysis faster than adding too much salt ruins a dish.
Strategies to Manage Missing Values Effectively
Facing missing values? You've got options: input missing values based on educated guesses or scrap them altogether, kind of like deciding whether to patch up jeans or buy new ones. But be careful; incorrect assumptions might lead you down the wrong path quicker than bad GPS directions.
Eliminating Duplicate Entries to Enhance Dataset Accuracy
Duplicate entries are more unwelcome than double text messages—they clutter your dataset and confuse algorithms. By using advanced deduplication methods such as Address Verification System (AVS), you remove unwanted echoes in your data, ensuring each point sings its own tune clearly within the symphony of information at hand.
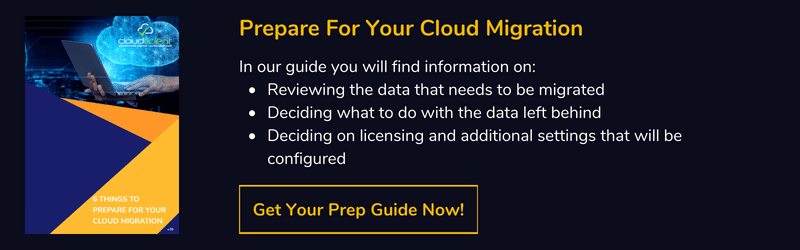
Step-by-Step Guide to Cleaning Your Data
Ever felt like a detective sifting through clues when you clean data? It's pretty much the same, except your suspects are duplicate entries and irrelevant observations. The first step is to round up these usual suspects by identifying them—this means removing any data that doesn't relate directly to your analysis.
The next part of our cleaning process feels like playing matchmaker, but instead of setting people up on dates, we're finding and fixing missing values in our dataset. Sometimes it’s about filling gaps with educated guesses—a technique known as imputation—or deciding they’re just not meant to be and deleting them altogether.
Last but not least, say goodbye to those pesky doppelgängers cluttering your data set. By using de-duplication tools, you'll merge multiple instances into one golden record for accuracy's sake. After all this work, remember: clean data leads us down the path of pristine decision-making—minus the drama.
The Critical Role of Clean Data in Strategic Decision-Making
Imagine trying to nail a bullseye with a dart while blindfolded—that's decision-making with dirty data. It's a shot in the dark, and it can cost companies big time. But when you've got clean data? You're taking that shot with laser precision.
Clean data doesn't just mean scrubbing away duplicates or tossing out null values like last week’s leftovers; it involves ensuring every bit of your information is accurate and relevant. Think about it—dirty data leads to false conclusions, which aren't as useful for business strategy.
And here’s where things get spicy: Ensuring pristine datasets isn’t just some mundane task; it's an art form—a dance between man and machine where cleaning tools twirl through rows upon rows of entries, correcting errors left by human oversight or faulty systems. We're not just removing unwanted outliers but shaping the very foundation upon which future empires can be built—or crumble into dust because someone forgot to check their naming conventions.
Advanced Techniques for Ensuring Pristine Datasets
Clean data isn't just nice to have; it's a powerhouse behind smart decision-making. But let's face it, achieving pristine datasets is like trying to keep your white sneakers clean in a mud run—it requires some serious effort and the right tools.
Enter all-in-one solutions for comprehensive data cleaning—think of them as the Swiss Army knife in your data toolkit. These platforms streamline workflows, letting you tackle everything from removing unwanted outliers to fixing mislabeled categories with finesse. They're kind of like that friend who organizes your closet and somehow makes everything fit perfectly.
Diving deeper into techniques, imagine playing whack-a-mole with duplicate entries or hunting down those pesky structural errors—the thrill. With automated workflows provided by modern cleaning tools such as Domo, Yellowfin BI, and Wyn Enterprise, these tasks become less about guesswork and more about precision strikes. Now that’s what I call hitting the bullseye for quality data.
The Future Landscape of Data Cleansing Technology
Imagine a world where data cleaning is as smart as your morning coffee maker, brewing up quality data while you tackle other tasks. That's the future we're looking at with the integration of artificial intelligence in data cleaning. Trends are showing us that AI and machine learning aren't just buzzwords; they're game-changers set to turbocharge automated algorithms for efficient cleansing.
Data scientists once spent hours, if not days, wrestling with dirty data—think incorrectly formatted entries or duplicate records spread across multiple departments. But now, cutting-edge AI tools swoop in to scrape away those unwanted outliers and mislabeled categories like a pro cleaner tackling a well-used kitchen.
What does this mean for organizations? They can kiss goodbye to the tedious task of manually sifting through endless rows of incomplete entries and null values. With advanced systems predicting when an outlier exists or when naming conventions don't match up across different datasets, businesses are poised to let these smart solutions take the wheel on their journey toward pristine datasets—and decision-making powered by nothing but clean facts and figures becomes reality.
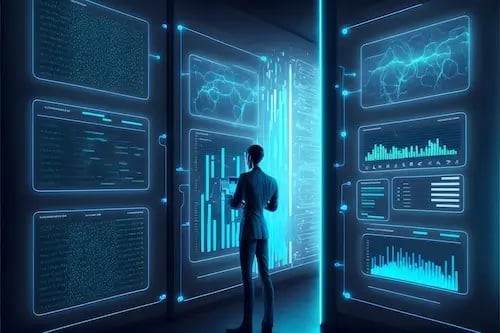
Conclusion
So you've explored what is data cleansing and why it's crucial for making sharp business decisions. You've learned to spot the sneaky errors that can throw your data off balance, from missing values to incomplete data to duplicates.
You know now how a solid cleaning process turns raw numbers into trustworthy insights. You’ve seen firsthand how clean data cuts through the noise, guiding businesses toward smarter strategies. Data cleaning, while not the most glamorous task in a business is essential.
And remember: scrubbing away irrelevant details does more than just polish up figures—it carves out a path for precision in data analytics.
The journey doesn't end here. With these takeaways in hand, you're set to foster datasets that are as pristine as they are powerful—fueling decisions with confidence and clarity.
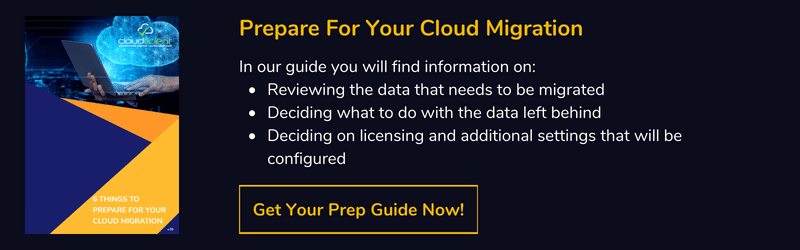