Excellent data leads to excellent business decisions. However, though this is common knowledge, only 3% of corporate data meets quality standards, according to a 2017 Harvard Business Review report and reconfirmed by Thomas C. Redman, one author of the initial report, in a 2020 update. Nearly 40% of business objectives fail because of bad data, costing businesses and the U.S. a collective $3 trillion.
Thankfully, companies have a solution to the poor data dilemma. What is data profiling, and how does it help?
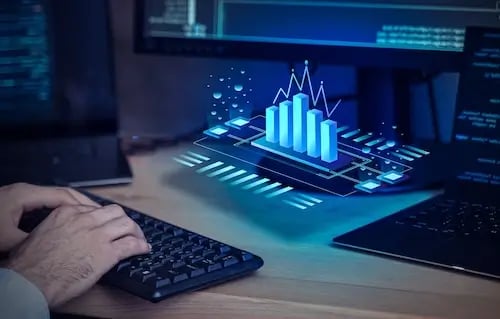
What Is Data Profiling and Why Is It Important?
Typical business operations produce enormous amounts of data. The information is diverse, involving accounting and finance, business spending, customer purchase histories, and other operating metrics. The data profiling definition comprises reviewing and analyzing existing datasets across a business to pull valuable and useful data to the forefront rather than letting it get lost in the ever-expanding virtual filing cabinet.
The ultimate purpose of profiling is to establish a useful and navigable inventory of business data, helping to improve the quality of business intelligence gathering and decision-making. With improved datasets, a company stands to make more profitable decisions while reducing costs and improving efficiency, building profits in the long term.
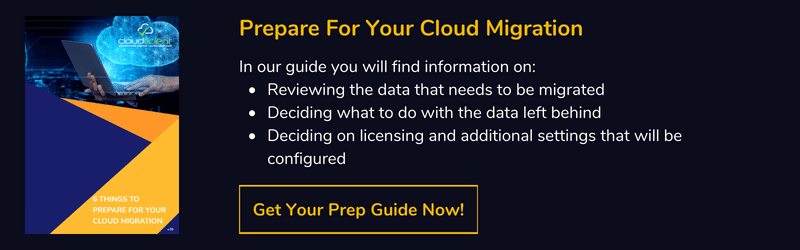
How Do Companies Profile Data?
The profiling process includes the examination and cleansing of existing datasets to purify information. The goal is to eliminate redundancies and corruption within datasets and systems, leaving only clean and useful information behind. Companies can use one or a combination of three data profiling techniques: manual, automated, or expert.
Manual Data Profiling
Manual methods are time-consuming and likely among the most expensive forms. This typically involves using a team of in-house professionals to scour all data to create a list and prioritize everything. The primary benefit of manual profiling is the advantage of human insight; however, with human insight comes human error.
Automated Data Profiling
Automated data profiling uses software and systems to speed up the process. Modern data profiling examples include the use of AI and machine learning to navigate information systems and interpret data quality. Most businesses use a combination of manual and automated profiling to make the process affordable and efficient.
Expert Data Profiling
Finally, expert data profiling involves outsourcing. Many businesses do not have the in-house experts or capabilities to handle comprehensive profiling. These companies hire a data profiling service to analyze, compile, and clean their data, paying a premium.
Most companies favor a multi-pronged approach. By combining two or more methods, a company ensures the cleanest resulting dataset, allowing for the most informed business decisions. Unfortunately, combining techniques can mean increasing the costs of the process.
What Are the 3 Types of Data Profiling?
Data profiling breaks down into three distinct discovery categories: structure, content, and relationship discovery. Each category helps data engineers establish a measurement and expectation for quality data.
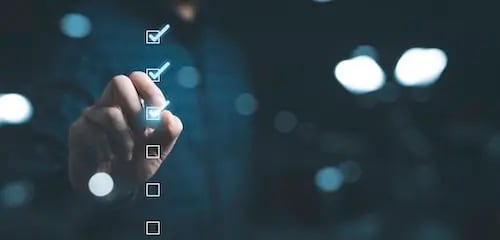
1. Structure Discovery
Structure discovery or analysis is a validation process. The purpose is to locate and compare datasets to ensure proper formatting. If a company wants accessible and searchable data, it needs to ensure that all appropriate fields correlate with relatable information.
The discovery process uses basic statistics, such as sum, minimum, and maximum, to validate data. It can also use descriptive statistics to review data consistency and structure. One core approach to the method is pattern matching, where data engineers use known patterns within specific data to test records against. For example, if a database has a column for client contact numbers, structure discovery can identify the percentage of the data that is missing the correct number of digits for a phone number.
2. Content Discovery
Content discovery examines individual records for quality and errors. The process can identify the rows in a dataset that contain problems or systemic inaccuracies. For example, using the same phone number example, content discovery could identify the percentage of phone numbers missing an area code.
3. Relationship Discovery
Relationship discovery is a more integrated and revealing process that involves examining data to identify relationships between various datasets. This form of profiling is critical to business decisions because it can help companies streamline marketing efforts or create a more targeted approach to manufacturing and distribution. As with structure and content discovery, relationship discovery is about finding patterns or relatable information, but it takes the process a step further by looking for overlap across all datasets.
While structure, content, and relationship discovery are all distinct arms of data profiling, a company makes the most of the process when it combines all three methods to clean, organize, and reaffirm data.
What Are the Benefits of Data Profiling?
Data profiling produces a multitude of critical benefits, from improved data quality to risk mitigation. Using formal methods for cleaning, approaching, and interpreting information makes for more useful data, leading to more informed decisions and assessments. By profiling datasets, a company gains insight into the quality, accuracy, consistency, and reliability of its information assets.
Enhanced Business Decision-Making
Understanding data quality and mitigating any issues is crucial to business decision-making, compliance, customer service, and profitability. Anomalies or errors in data can compromise operations and reporting, resulting in distribution errors, potential regulatory hiccups, and revenue loss.
Creation of Data Models and System Integrations
Beyond risk mitigation and data governance, data profiling helps in designing quality data models, incorporating and executing ETL processes, and making further integrations. In a nutshell, it can perpetuate data democratization and aid in creating centralized information.
The goal of any information platform should be usability. People across an organization should know how to find and retrieve the data necessary for decisions. Profiling data supports this to empower users to work efficiently.
Security Improvements and Vulnerability Detection
Data profiling can lead to security improvements. The process helps organizations identify vulnerabilities within the data storage systems or patterns within user activity. In financial institutions, profiling can help identify fraudulent transactions or highlight areas for improvement in fraud detection.
Better Marketing and Customer Service
Many companies focus on the internal benefits and in-house advantages like cost savings and efficiency improvements, but data profiling offers so much more.
Better consumer insights allow businesses to improve marketing and advertising efforts and customer service. For example, reviewing customer data can highlight the specific needs and preferences of clients, allowing a business to create accurate consumer profiles and increase personalization. This in turn can improve customer experiences and increase retention rates.
For example, with the right, well-organized data, companies can identify the average churn rate and timeline for clients. By understanding this number and timeline, businesses can target clients reaching the end of the cycle to entice them to stay.
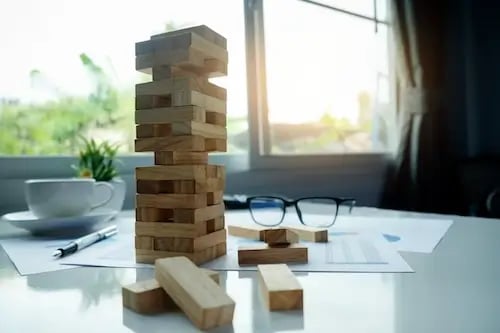
What Are the Challenges of Data Profiling?
Data profiling is essential to the creation and maintenance of profitable data architecture. Unfortunately, several common challenges and pitfalls can interfere with the process and its accuracy.
Quality Control Issues
One of the primary challenges of data profiling is quality issues. Duplicate, inaccurate, inconsistent, or missing data can interfere with the profiling process. It can skew reporting and affect profiling results. Using data quality tools can help automate the detection and correction of quality errors during manual discovery.
Diversity and Complexity of Datasets
Business leaders may incorrectly assume data profiling is about simplifying datasets. In fact, the primary goal is not simplification in itself but rather accessibility and interpretation.
A significant problem in profiling is the diversity and complexity of data. Many companies use many platforms, systems, and formats with different semantics and schemas to collect data. To overcome this challenge, data engineers need tools and software capable of handling multiple types of datasets, such as structured, unstructured, semi-structured, and streaming. Data mapping and transformation techniques are also necessary to convert data and make it more usable in the corporate landscape.
If a company wishes to use data profiling to assess its data, it first needs to have its data in one place. It is nearly impossible to profile data that exists across several departments and incorporates multiple storage solutions or types. Companies should focus on compiling data in a centralized location and onboarding a data professional to help manage the information.
Expense and Time
The initial setup phase for data profiling makes it cost-prohibitive for many companies. In addition, the expense of the process overall is a barrier for small to medium-sized businesses. Data profiling is time-consuming and complex. The sheer volume of data produced by a business in any year means a profiling program faces challenges from the start.
Still, it is possible to reduce the costs and time allotment for the process. Companies have marketplace tools and software that can aid in data migration and integration. Moreover, data profiling often proves its return on investment when companies compare the potential profit losses related to bad data.
How Can Cloudficient Help in the Data Profiling Process?
Understanding the data profiling meaning is the first step toward implementation. While some companies may struggle with the potential expenses of this process, they must weigh those costs against the potential losses without it. There are also ways to reduce the initial and ongoing costs of profiling, including the use of data migration software and incorporating automation software.
The answer to the question of what is data profiling is simple: It is a process for cleaning and ensuring the quality of information. However, its affordable integration may seem more difficult — that is, until you consider Cloudficient, a service and tool for data migration, intelligent retention, and streamlining of datasets. When you are ready to make the most of your data and improve its usefulness to your business objectives and decisions, contact a Cloudficient representative to learn more about how we can help with your business's data profiling needs.
With unmatched next generation migration technology, Cloudficient is revolutionizing the way businesses retire legacy systems and transform their organization into the cloud. Our business constantly remains focused on client needs and creating product offerings that match them. We provide affordable services that are scalable, fast and seamless.
If you would like to learn more about how to bring Cloudficiency to your migration project, visit our website, or contact us.
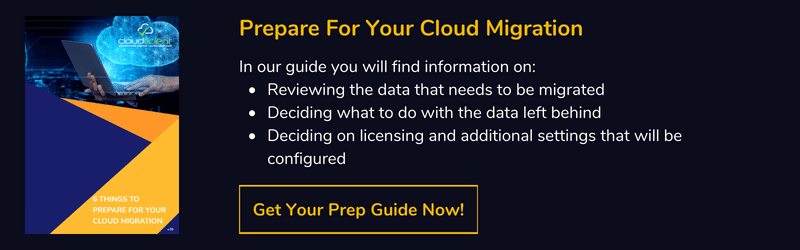