Imagine standing on the edge of a grand canyon, data conversion in one hand and data migration in the other. Each represents a distinct journey across the data landscape but understanding which path to tread can be daunting. Data conversion vs data migration, what are the differences?
Have you ever been in a similar situation? Lost in translation between databases or migrating precious data from one location to another only for it to become as indecipherable as hieroglyphics. These are not just processes; they're adventures that can make or break your business' future.
We're about to delve deeper into the complex world of data conversion vs data migration. We'll uncover the nuances, tackle challenges head-on, and learn from best practices. This knowledge will be your secret weapon for smooth conversions and migrations. So buckle up! It's time we navigate this intricate landscape together.
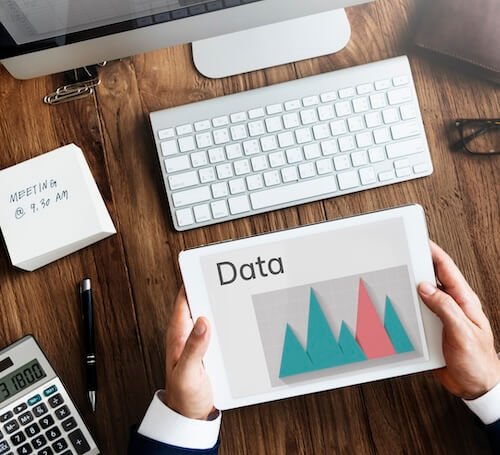
Table Of Contents:
Understanding Data Conversion vs Data Migration
Data conversion and data migration are two pivotal aspects of data management. Although they sound similar, these processes serve distinct purposes. Data quality, is fundamental to both concepts.
Data conversion, as its name suggests, involves changing the format of your data. This process is like translating a language; you take information in one form (or 'language') and transform it into another that's understood by your target system. Think about converting a Word document to a PDF file—it’s the same content but in different formats. Some data types can be interchangeable depending on the use of the data.
You can learn more about this from Wikipedia's explanation on data conversion.
Data conversion tools exist to help manage the data conversion process. For some organizations, time and effort related to conversion might mean they investigate outsourcing data conversion to meet project timelines.
In contrast, data migration focuses on moving existing datasets from one location or system to another—similar to relocating homes. You're not changing what the data says (like with conversions), but where it resides.
To delve deeper into data migrations, check out our detailed article.
It's worth noting that data cleansing is sometimes undertaken at the same time as data transformation of either of these kinds.
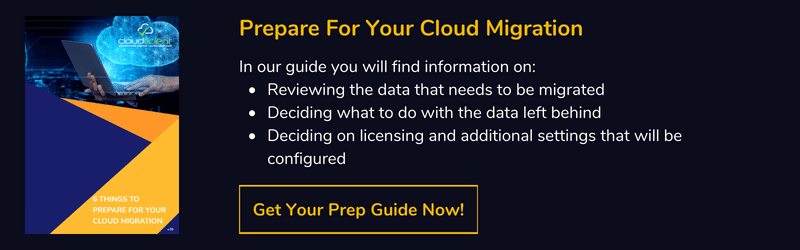
The Interplay Between Conversion and Migration
Sometimes these processes intersect—for instance, when migrating between systems that handle different data formats. In such cases, we might need both: convert our documents then migrate them over.
Key Differences Between Data Conversion and Data Migration
Understanding their differences can help make your data management more efficient.
Defining the Conversion Process
The process of data conversion is about transforming existing data into a new format that's compatible with your target system. It involves complex processes like identifying different types of source data, mapping each element to its corresponding place in the new system, and using APIs or ETL tools for converting it. Ensuring quality during this step is vital because incorrect conversions can lead to inaccurate or lost information.
Data mapping can be a painstaking process, and will, after the conversion usually also involve some data validation processes. This is to ensure that the data is still available to be used by end systems. Sometimes rather than validating all data, certain key data sets will be taken and validated.
Understanding the Migration Process
Data transfer, instead of conversion, concentrates on transporting datasets from one spot to another without altering their form or structure. This typically requires less time than conversions unless additional transformations are needed. Careful planning before migrating helps prevent common pitfalls such as alignment issues between systems.
In summary, while both involve handling vast amounts of information effectively, each has unique characteristics requiring specific tools and approaches for success.
Factors to Consider in Data Conversion and Data Migration
Data conversion and migration may seem simple, but they're complex processes that require careful planning. Let's dig into some factors affecting these projects.
Quality Assurance in Conversion and Migration
Maintaining data integrity during conversion or migration is crucial. It's not just about moving or converting data; it’s about assuring its quality too. Remember, inaccurate data can cause serious issues post-migration. The conversion process involves assessing types of the existing dataset, identifying an API for conversion while ensuring accurate results.
You need to identify each element being moved in a migration project as well. Mapping each element correctly ensures minimal risk of data loss.
Importance of Data Governance
Data governance plays a pivotal role here as well - making sure all source formats are compatible with destination systems saves time during database migrations.
In essence, both processes require due diligence when handling source format datasets to ensure successful conversions follow through without compromising on the competitive advantage gained from your stored information. Remember: Decisions based on good-quality converted or migrated data drive success.
Challenges in Data Conversion and Data Migration
Two of the most common problems faced during these processes involve alignment requirements and file format issues.
Addressing Alignment Requirements
The first hurdle is making sure that your source data aligns with the target system's specifications. Navigating alignment issues can be difficult, especially when transferring large datasets or different types of data; without proper alignment, the integrity of the data may be compromised and result in losses or inaccuracies. Without proper alignment, you risk compromising data integrity, leading to potential losses or inaccuracies.
A second significant challenge comes from managing multiple file formats. When converting or migrating data, it's not uncommon to encounter a range of formats - everything from flat files to complex database structures. It’s like trying to fit square pegs into round holes. So understanding how each format works helps ensure smooth sailing through this tricky process.
Addressing these issues head-on reduces the likelihood of stumbling blocks during your conversion or migration project while improving overall outcomes for accurate data management.
Best Practices for Successful Data Conversion and Data Migration
Migrating or converting data is a delicate task. The key to success? A solid plan, the right tools, and an unwavering focus on quality.
Planning for Success
The first step in any successful data conversion or migration project is careful planning. You need to understand your source format, target systems, and all types of data involved. This will help you anticipate challenges like misaligned data fields or incompatible formats.
An effective plan also involves mapping out each stage of the process - from extracting source data to loading it into the destination system - which helps minimize risks such as inaccurate conversions and potential data loss scenarios.
Selecting suitable migration tools and conversion tools is another critical factor.
The best ones facilitate easy transformation of various types of existing datasets while ensuring high-quality results with minimal errors. They can even automate certain parts of the process – reducing manual effort, improving efficiency.
Take a look at our suite of products and solutions based on our scalable ReMAD platform.
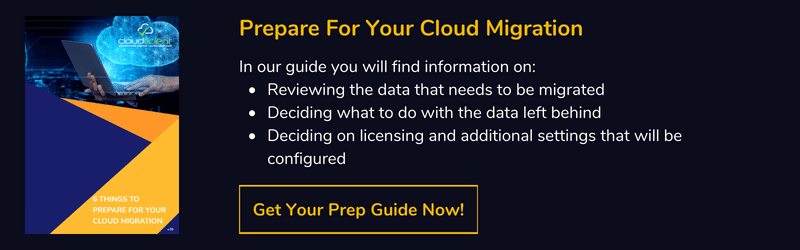
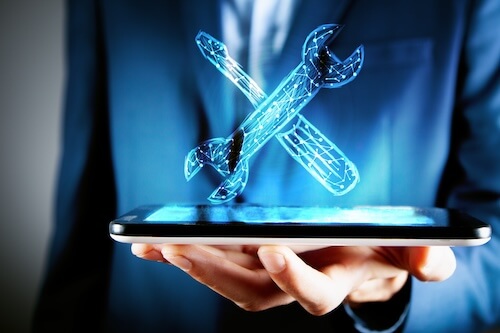
Conclusion
Unraveling the complexities of data conversion vs data migration can feel like a monumental task. But you've done it!
You've delved into the unique characteristics and processes that make up each, understanding their distinct roles in successful data management.
Data conversion is not just about changing formats; it's also about ensuring compatibility with new systems. Data migration, on the other hand, is more than moving datasets; it requires effective strategies to avoid common pitfalls.
We've tackled challenges together and identified best practices for smoother transitions during both conversions and migrations. Armed with this knowledge, your next adventure across the intricate landscape of data should be less daunting.
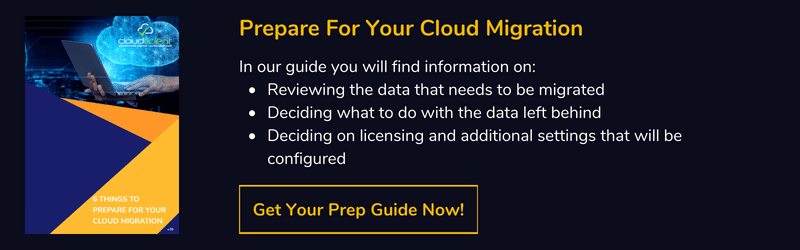